21.1A : Trustworthy Sustainment Solutions via a Learning-Assisted Digital Twin
PointPro, Inc. offers a computational platform that performs adaptive, closed-loop predictive simulations in order to support the Air Force in its sustainment operations. The future of sustainment is in prescriptive analytics: predicting what will fail, when & how it will fail, and, what can be done about it?
Prescriptive analytics demands timely, accurate forecasts of a system’s path to failure. PointPro builds on the user’s digital twin physics-based models to deliver forecasts within user defined accuracy. Our software leverages the time-tested robustness and versatility of Monte Carlo simulations in a closed-loop architecture, making it possible to deliver guaranteed accuracy in the forecast of system specific quantities of interest. When prediction errors are detected to exceed user-defined bounds, a sequence of optimization problems are solved, that in effect answer a long-standing question in predictive simulation: to meet a required level of accuracy, how big should the simulation size be? Consequently, a decision-quality, certifiably actionable forecast is generated in a single-shot, in minimal time. The platform integrates equally with physics-based evolutionary models and with data-driven (ML) models; and offers means to improve such models. In benchmark studies conducted so far, up to 3x productivity gain has been demonstrated. Moreover, gains improve with increasing system complexity.
In this Phase I STTR, a feasibility study is proposed to elicit feedback from SMEs regarding PointPro use for forecasting complex systems. In particular, the project will focus on optimized asset sustainment needs for USAF jet engines and space assets. We intend to support existing predictive maintenance needs and accelerate prescriptive maintenance capabilities. Feedback related to specific users, applications, and necessary additions to the software will serve as a workplan for further work.
21.1A : The Smart Self-Aware Manufacturing Machine (SSAMM)
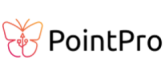
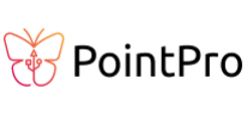